|
Elena Pugacheva
Complexity of Financial Crisis
Abstract
In this paper the ideas of the theory of complexity as to the world financial crisis is discussed. Some systemic weaknesses of the traditional approach is considered. The potential of the theory of complexity in searching the ways of financial crisis overcoming is outlined. The peculiarities of financial crisis in Ukraine from the complexity point of view are shown.
Keywords: complexity, self-organization, network, financial crisis
1. Introduction
Our world becomes more complex every day. To cope with it, the systems we design and control also need to become more complex increasing the complexity of the world. In recent decades, the study of complex systems has been transforming the way we view our world (Anderson, P.W. (1972); Bar-Yam, Yaneer (1997); Вarabasi, Albert-Laszlo (1999); Kauffman, Stuart (1993)). Today there are some very famous for their scientific results centres for complex systems: Santa Fe Institute (New Mexico, USA,1984 ), New England Complex Systems Institute (USA,1996), Complex systems Society (2004).
There are many ways to describe socio-economic system. Every scientific approach has its own context in the framework of which it becomes more appropriative. The theory of complex system doesn’t deny any of existing approaches. It is complementary approach that becomes useful when the system or environment is very dynamic and/or unpredictable.
2. Complexity as a new way of thinking
Nowadays it is very important to understand that we live not only in the time of world financial crisis but also in the time of huge scientific shift that should influence all aspects of our life. The problem is that world changed drastically and our model of the world should be defined more exactly. Complexity and interdependence are the key challenges of our time. Coping with complexity is the problem that could not be decided in the framework of our traditional way of thinking. “Classical” approaches are still very useful, but only for problems in stationary state. But with an increase of complexity, problem domains become non-stationary, requiring for dynamic solutions that will be able to adaptto the changes in the problem domain (Ashby, 1947a). Complex behavior arises through the interrelationship, connectivity and interdependence of different entities (individuals, organizations, institutions, etc.) and their feedback processes (positive and negative).
W. Brian Arthur is one of the early economic researchers in the field complexity. Arthur's comments on the complexity theory as a different way of seeing and conducting scientific inquiry: “Conventional economic theory chooses not to study the unfolding of the patterns its agents create, but rather to simplify its questions in order to seek analytical solutions. Thus it asks what behavioral elements (actions, strategies, expectations) are consistentwith the aggregate patterns these behavioral elements co-create? For example, general equilibrium theory asks: what prices and quantities of goods produced and consumed are consistent with—would pose no incentives for change to—the overall pattern of prices and quantities in the economy’s markets. Game theory asks: what strategies, moves, or allocations are consistent with—would induce no further reactions to—the potential outcomes these strategies, moves, allocations might imply. Rational expectations economics asks: what forecasts (or expectations) are consistent with—are on average validated by—the outcomes these forecasts and expectations together create. Conventional economics thus studies consistent patterns—patterns in behavioral equilibrium, patterns that would induce no further reaction. Economists at the Santa Fe Institute, Stanford, MIT, Chicago, and other institutions, are now broadening this equilibrium approach by turning to the question of how actions, strategies, or expectations might react in general to—might endogenously change with—the aggregate patterns these create. The result, complexity economics, is not an adjunct to standard economic theory, but theory at a more general, out-of-equilibrium level…Complexity therefore portrays the economy not as deterministic, predictable and mechanistic; but as process-dependent, organic and always evolving” [4].
3. Some core ideas of the theory of complexity
Today economists are open to a great deal of extend not to mechanical but to the organic approach. In the framework of organic approach economics is seen as self-organizing complex system [1], economic environment is perceived as ecosystem [24] and the problem of economic growth is interpreted as the problem of co-evolution of economic system with its environment [18, 10].
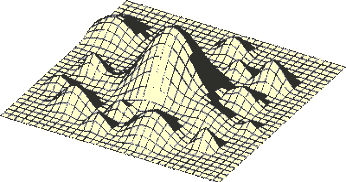 |
Fig.1 «Fitness Landscape» Business environment will have a 'rugged' adaptive landscape with many peaks that are constantly changing |
The model «Fitness Landscape», proposed by Stuart Kauffman [20], could be a good illustration of the idea of complex environment. As soon as landscape has been changing all the time your attempts to climb to the top of the hill could be fruitless not because of your own mistakes and faults but because the landscape at some moment could shift down independently of your activities. At the same time the web of connections could reinforce not only risks but the opportunities for grows. As Brian Arthur’s researches show fundamental law of mainstream economic theory “Law of Diminishing Returns” is not always true in web-structure. Quite the contrary, the “Law of Increasing Returns” often take place. Arthur explains that: 'If a product or company or a technology-one of many competing in a market- gets ahead by chance or clever strategy, increasing returns can magnify this advantage and the product or company or technology can go on to lock in the market' [3]. So even the inferior product could survive and thrive because it is linked to many other products in the community and thus excludes competitors.
The model of behavior is also changed in web-structure. Thus whereas in traditional business thinking, based on head-to-head competition the aim is a win/lose situation ('I win , my competitor loses') in the more complex co-evolutionary business environment the aim is a win/win situation in the knowledge the business will succeed if others do. Under the conditions of turbulent fast changing environment it is necessary to make strategic decisions to survive. A more appropriate and powerful approach requires thinking in terms of whole systems, that is web-thinking. Web-strategy asserts that the two basic choices confronting senior management are which webs [or ecosystems] to participate in (or to form), and what role to play in them [25].
Today social networks are growing rapidly. And what is more important they are growing without central control. The structure and dynamics of social networks are determined by interactions between the participants, who, in turn, often have opposite interests. Last researches in the field of social networks make it possible to see the architecture of the order that is constructed by the processes of self-organization [12]. Besides, network theory offers a successful conceptual framework to approach the structure of many complex systems.
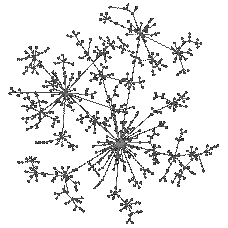 |
Fig.2 The model of preferential attachment More connected node continue to be in high demand high demand |
The scenario of network growth could be schematically described such a way (Fig.2): any new participant join network but not in random manner (according to random attachmentmodel). The point is that different participants have different number of connections. Some of them have only a few connections, but some of them (small part) have hundreds and operate as hubs. Indeed, the more connections the participant of social network has, the higher chances to be found by newcomer. The probability that it will choose a given node is proportional to the number of links the chosen node has. That is, given the choice between two nodes, one with twice as many links as the other, it is twice as likely that the new node will connect to the more connected node. So with more higher probability newcomer will join some hub. This process is called the model of preferential attachment. The dynamics of social network growth could be described as rich-get-richer phenomenon in which more connected node continue to be in high demand. It is interesting to note that the fight for links are not antagonistic, nodes with different number of links coexist peaceful. This means that the interactions form networks, where each node interacts with only a small number of selected partners whose presence and effects might be felt by far away nodes.
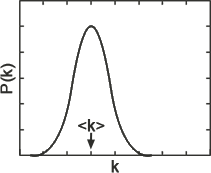 | 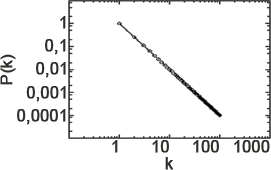 |
Fig.3 Poisson distribution (bell curve) and “power law” distribution |
Statistic characteristics of self-organizing network differs from corresponding characteristics of random system. First of all the probability that a node is connected to k other nodes follows not a Poisson distribution (bell curve) but “power law” distribution (Fig.3). The most important difference between these two laws is that probability of significant deviations is much higher. Hubs make the network robust against accidental failures but vulnerable to coordinated attacks. The removal of a node with a small degree does not significantly disrupt the network topology. Moreover with up to 80% of the nodes of a scale-free network removed, the remaining nodes remain part of a compact cluster. At the same time such a network fails when the central hub is removed. The structure of scale-free networks help to explain spread of computer viruses, diseases, economic crash.
The above scientific results signify shift in thinking: from static to growing and from structure to evolution. It is necessary to realize that we live in closely connected by social links world, where the consequences of our actions have nonlinear character. Advantages and shortcomings of interconnected systems are obvious. It is possible to get unprecedented benefits due to the economics of increasing returns. At the same time changes in one part of the system could damage you because you are tightly connected with another economic agents by means of intricate web of links. The nature of modern social network is the complex combination of risks and opportunities. Networks reinforce risks but at the same time multiply the possibilities of technical innovations and lower the level of system complexity for individuals. As links and connections take over understanding of network effects become the key to survival in a rapidly evolving new economy.
For years, economists spoke of formal model of economics, in which companies interact not with each other but with the market. As Albert-Laszlo Barabasi said: “In reality, the market is nothing but a directed network. Companies, firms, corporations, financial institutions, governments, and all potential economic players are the nodes. Links quantify various interactions between these institutions, involving purchases and sales, joint research and marketing projects, and so forth. The weightof the links captures the value of the transaction, and the direction points from the provider to the receiver. The structure and evolution of this weighted and directed network determine the outcome of all macroeconomic processes”.
So the state of economy is determined as the result of everyday local interactions of economic agents and the result of such relations could not be obtained as a direct sum of individual actions. It would be very great oversimplification to suppose that all economic agents have the same feelings, thoughts, goals, reactions, etc.
It is not coincidence that the tendency of social modelling is the transition from factors to actors. In the 1960s, the first wave of innovation used computers to simulate control and feedback processes in organizations, industries, cities, and even global populations. In the 1970s, the second wave of computer modellers introduced the use of individuals as the units of analysis but retained the earlier emphasis on empirically based macro-level forecasting. In the 1980s, the third wave in social simulation distinctive characteristic of which is that individuals are not socially isolated actors but agents that interact interdependently.
Unlike earlier approaches to computer simulation, whose value depends largely on predictive accuracy, agent-based models are “much more concerned with theoretical development and explanation than with prediction” (Gilbert 1997, p. 2.1). "... the goal of agent-based modelling is to enrich our understanding of fundamental processes that may appear in a variety of applications" (Axelrod 1997, p. 25).
4. Complexity Economics versus Traditional Economics
Brian Arthur, Steven N. Durlauf, and David A. Lane, of the Santa Fe Institute define six features of complex [7]:
- Dispersed Interaction — What happens in the economy is determined by the interaction of many dispersed, possibly heterogeneous, agents acting in parallel. The action of any given agent depends upon the anticipated actions of a limited number of other agents and on the aggregate state these agents co-create.
- No Global Controller — No global entity controls interactions. Instead, controls are provided by mechanisms of competition and coordination between agents. Economic actions are mediated by legal institutions, assigned roles, and shifting associations. Nor is there a universal competitor—a single agent that can exploit all opportunities in the economy.
- Cross-cutting Hierarchical Organization — The economy has many levels of organization and interaction. Units at any given level behaviours, actions, strategies, products typically serve as "building blocks" for constructing units at the next higher level. The overall organization is more than hierarchical, with many sorts of tangling interactions (associations, channels of communication) across levels.
- Continual Adaptation — Behaviours, actions, strategies, and products are revised continually as the individual agents accumulate experience—the system constantly adapts.
- Perpetual Novelty Niches — These are continually created by new markets, new technologies, new behaviours, new institutions. The very act of filling a niche may provide new niches. The result is ongoing, perpetual novelty.
- Out-of-Equilibrium Dynamics — Because new niches, new potentials, new possibilities, are continually created, the economy operates far from any optimum or global equilibrium. Improvements are always possible and indeed occur regularly.
The table below illustrates the differences between the complexity perspective and classical economics [16].
|
Complexity Economics |
Traditional Economics |
Dynamic |
Open, dynamic, non-linear systems, far from equilibrium |
Closed, static, linear systems in equilibrium |
Agents |
Modelled individually; use inductive rules of thumb to make decisions; have incomplete information; are subject to errors and biases; learn to adapt over time; heterogeneous agents |
Modelled collectively; use complex deductive calculations to make decisions; have complete information; make no errors and have no biases; have no need for learning or adaptation (are already perfect), mostly homogeneous agents |
Networks |
Explicitly model bi-lateral interactions between individual agents; networks of relationships change over time |
Assume agents only interact indirectly through market mechanisms (e.g. auctions) |
Emergence |
No distinction between micro/macro economics; macro patterns are emergent result of micro level behaviours and interactions. |
Micro-and macroeconomics remain separate disciplines |
Evolution |
The evolutionary process of differentiation, selection and amplification provides the system with novelty and is responsible for its growth in order and complexity |
No mechanism for endogenously creating novelty, or growth in order and complexity |
Technology |
Technology fluid, endogenous to the system |
Technology as given or selected on economic basis |
Preferences |
Formulation of preferences becomes central; individuals not necessarily selfish |
Preferences given; Individuals selfish |
Origins from Physical Sciences |
Based on Biology (structure, pattern, self-organized, life cycle) |
Based on 19th-century physics (equilibrium, stability, deterministic dynamics) |
Elements |
Patterns and Possibilities |
Price and Quantity |
5. Complex Theory about Financial Bubbles
The important conclusion from Cagan’s analysis (Cagan 1956) was that, in hyper-inflated economies, price variations are larger than real variables variations. So general price could be written as the sum of fundamental price (associated with the fundamental of the economy, i.e., monetary balance) and second component (associated with agents’ expectations of the levels of future prices, referred to as “bubble”), i.e. ptg = ptf +bt. Thus, the general price depends on monetary policy and the agents’ expectations of prices.
W. Brian Arthur gives the following explanation to the “anomalies” such as unexpected price bubbles and crashes, random price variation [4]. The standard theories of financial markets are based on the model of rational expectations [21]. It assumes that agents can somehow deduce in advance what model will work, and that everyone “knows” that everyone knows to use this model (the common knowledge assumption). The question is: “What happens when forecasting models are not obvious and must be formed individually by agents who are not privy to the expectations of others?”.
Arthur, Holland, LeBaron and Palmer [6] have created a model which relaxes rational expectations by assuming that investors cannot assume or deduce expectations but must discover them. Agents continually create and use multiple "market hypotheses"—individual, subjective, expectational models—of future prices and dividends within an artificial stock market on the computer. These “investors” are individual, artificially-intelligent computer programs that can generate and discard expectational “hypotheses,” and make bids or offers based on their currently most accurate hypothesis. The stock price forms from their bids and offers, and thus ultimately from agents’ expectations. So this market-in-the-machine is its own self-contained, artificial financial world. The expectations compete in a world these expectations create.
Within this computerized market, two phases or regimes were found. If parameters are set so that artificial agents update their hypotheses slowly, the diversity of expectations collapses quickly into homogeneous rational expectations ones. The reason is that if a majority of investors believes something close to the rational expectations forecast, then resulting prices will validate it, and deviant or mutant predictions that arise in the population of expectational models will be rendered inaccurate. Standard finance theory, under these special circumstances, is upheld. But if the rate of updating of hypotheses is turned up, the market undergoes a phase transition into a “complex regime” and displays several of the “anomalies” observed in real markets. It develops a rich “psychology” of divergent beliefs that don’t converge over time. Expectational rules such as “If the market is trending up, predict a 1% price rise” that appear randomly in the population of hypotheses can become mutually reinforcing—if enough investors act on these, the price will indeed go up. Thus sub-populations of mutually reinforcing expectations arise, agents bet on these (therefore technical trading emerges) and this causes occasional bubbles and crashes. Artificial market also shows periods of high instability in prices followed randomly by periods of low instability. This is because if some investors “discover” new, profitable hypotheses, they change the market slightly, causing other investors to also change theirexpectations. Changes in beliefs therefore ripple through the market in avalanches of all sizes, causing periods of high and low volatility. In such situation actual financial markets lie in “complex” regime.
6. Complex Theory about Financial Crisis
Professor Yaneer Bar-Yam, president of New England Complex Systems Institute, considers that the source of the current problem is a lack of understanding about complex systems, namely ignoring interdependence – the increasingly strong web of connections between people and organizations [27]. Such powerful regulators as the Federal Reserve, the SEC, and Congress have failed to account for the increasingly strong connections among people and organizations. Bar-Yam complains: “Everyone knows that we are connected to each other, locally and globally. It is surprising, therefore, that this is not part of our economic policy, planning, or investment”.
Understanding of the nature of complex systems could help to avoid some mistakes and be careful with some usual practices the risk of which was underestimated. Bar-Yam says: “Remarkably enough, investors decide how to invest by considering the risk of each investment separately and adding together the total risk. But, since things are connected to each other, they cannot be evaluated independently.
Much of the economy works through positive feedback - if people buy more, more workers are hired, who in turn work more and earn more money to buy more goods. The problem with positive feedback loops is that they can run in the opposite direction: if people buy less, fewer workers are needed, which means lower earnings and less buying. What stabilizes these loops has to do with the many different ways and places people can work, buy and sell, and interact with the market. More variety creates greater stability in the system as a whole.
Danger arises when too many people are doing the same thing - as when too many investors buy certain stocks creating a stock market bubble; or when too many invest in mortgage-backed securities, leading to the bubble we have just experienced”.
Recognizing dependencies will lead to better regulation and a more stable market. It is essential to be aware of the appropriateness that when free markets become "too free," they become self-destructive. Professor Bar-Yam explains: “This is the old paradox of having too much of a good thing. Mortgages are a good idea, but making them too easy to get, or too hard to get, is not. Having too much of a good thing is a common economic problem, but regulators often fail to recognize it. Free markets are a good thing, but when they are unregulated (too free) then markets self-destruct.
If too much of anything is not good, how do we prevent it? Moderation is considered a personal virtue. Moderation is also an essential economic principle. What is bad for markets is when one player, or a coordinated group, controls the movement of prices. Regulations can restrict the extent of short selling, or rapid buying, rather than forbid or allow it”.
Yaneer Bar-Yam suggests to test economic policy as strictly as it usually take place in science. It hasn’t been a common practice yet, but contemporary scientific achievements could help to simulate complex situations. Complex systems modelling demonstrates how easy to believe in something that isn’t true in reality. The models that could help us to understand collective behaviour could prompt more effective managerial solutions. It is necessary to point out that economic science hasn’t devoted necessary attention to such ideas yet.
7. Peculiarities of Financial Crisis in Ukraine
Ukraine as a participant of world global economic system is involved in world financial crisis which has systemic character. But from the complexity point of view the main problems in Ukraine differ from the problems of the majority of developed countries. The main problem of the hubs of world economy, as was stated above, is a lack of understanding about complex systems. World economy should adequately respond to the increasing complexity of economic reality. It is very complicated problem but optimism in successful outcome lies in the fact that complexity of national economies are now rather high and it is possible under appropriative efforts to increase their complexity as the necessary condition of crisis overcoming.
As to Ukraine it is necessary to recognise that economic system has less level of complexity. Although there are no precise tools to measure the level of complexity it is quite clear that Ukrainian socio-economic system doesn’t have properties typical to the dynamics of complex systems. First of all, no one social institute evolves as network by means of preferential attachment. The links are mostly blood ties, friendship ties, unlawful. The second, all links are oriented in one direction. Very important for complex systems feedback mechanism is practically absent. And, the third, the people are not considered as agents of socio-economic systems. There is no link between the current and future state of the society and their activity. The goals of citizens of Ukraine are mostly material, pragmatic goals that more successfully could be achieved alone in severe competition struggle with fellow-citizens. So Ukrainian socio-economic system faced with world financial crisis has no protective mechanisms to resist its influences. The linear social structure doesn’t allow to multiply the possibilities and doesn’t lower the level of complexity for individuals.
The tight central control in such situation couldn’t resolve the systemic weaknesses. Hierarchy and central control imposes a limitation on the degree of complexity of collective behaviour and couldn’t increase the complexity of the whole system as integrity. Today the complexity of collective behaviour is higher that the complexity of individual. Once this is true hierarchical mechanisms are no longer able to impose the necessary coordination of individual behaviours. Instead, network interactions are necessary. Systems must enable its components to contribute effectively to collective system.
The problem is that the task to build adaptive socio-economic system has never been put forward in Ukraine. The necessity to prioritize this task hasn’t been realized yet. Moreover it requires another conceptual approach: to build adaptive systems involves designing the elements of a system to find themselves the solution of the problem, that is when the problem changes, the elements are able to dynamically find a new solution or self-organizes. From this point of view more efforts should be made on facilitation of improvements than on control of the system. It should be recognized that preparedness, sharing, trust, loyalty are very important to avoid the system failure.
Ukraine now is in the situation of oversimplification. Naturally, simplification is one of the possible ways to make system easier to regulate. But there is a limit to which systems can be simplified and if control models are oversimplified they cannot work. This limit could be described by Ashby’s Law of Requisite Variety (1956) “for appropriate regulation the variety in regulator must be equal to or greater than the variety in the system being regulated”. So oversimplifying may destroy complex systems or produce models that misrepresent the original system.
8. Conclusions
The main characteristics of contemporary world economy are: interrelationship, connectivity, interdependence, complexity, self-organization. Increased complexity is the result of growth interactions between organizations, agencies and individuals due to new communication technologies, erosion of the boundaries between countries, processes of globalizations, liberalization of economic activity. The processes of self-organizations are to great extent unpredictable and uncontrolled processes. The attempts to capsulate self-organization and impose control are not effective. The core of new paradigm is in interactions and networks that connect individual agents and other elements of economic system. From our point of view the main problem in current situation is the problem of choice [23]. It is the time to enable individuals to be the agents of self-organization. This decision is unprecedented in the sense that it needs distributed power and distributed control. It should be recognized that effectiveness of decision-making is a function of complex network activity effectively intertwined with bureaucratic structures that foster distributed leadership. It should be also recognized that control lies within complex dynamics not in bureaucracy. Today different countries have different level of preparedness for such conceptual transition but the search of the ways of today’s crisis overcoming draw nearer this ideas of complexity that for years were of interest of only specialized group of scientists.
References
- Anderson P.W., Arrow K., and Dines D., eds. (1988), The Economy as an Evolving Complex System. Santa Fe: Addison-Wesley Publishing Company, 9–28.
- Anderson, P.W. (1972), "More is Different: Broken Symmetry and the Nature of the Hierarchical Structure of Science", Science 177 (4047): 393-396
- Arthur W. B. (1996) “Increasing Returns and the Two Worlds of Business, Harvard Business Review, July-August.
- Arthur W. B. Complexity and the Economy Science, 2 April 1999, 284, 107-109.
- Arthur W. B., Complexity, 1 (1), 20, (1995).
- Arthur W. B., Holland J. H., LeBaron B., Palmer R., and Paul Tayler, in The Economy as an Evolving Complex System II, 15.
- Arthur; Durlauf, Steven; Lane, David A (1997), "Introduction: Process and Emergence in the Economy", The Economy as an Evolving Complex System II, Reading, Mass.: Addison-Wesley.
- Ashby, W.R. 1956, Introduction to Cybernetics, Chapman & Hall, 1956, p.124
- Ashby, W. R. (1947a). The nervous system as physical machine: With special reference to the origin of adaptive behavior. Mind 56 (221) (January): 44–59.
- Axelrod R. (1990), The Evolution of Cooperation, London: Penguin
- Axelrod R. 1997. The Complexity of Coopera tion. Princeton, NJ: Princeton Univ. Press
- Barabasi A.-L. 'The architecture of complexity', IEEE Control Systems Magazine 27:4, 33-42 (2007).
- Barabasi A.-L. Linked : the new science of networks, Perseus, 2002, p. 209.
- Barabasi, Albert-Laszlo; Hawoong Jeong & Reka Albert (1999), "The Diameter of the World Wide Web", Nature 401: 130-131.
- Cagan, P (1956), The monetary Dynamics of Hyperinflation, in Studies in the Quantity
- Colander, David. The Complexity Vision: The Teaching of Economics.
- Gilbert N. 1997. A simulation of the structure of academic science. Sociol. Res. Online 2(2):3.1-3.21.
- Hodgson G. M. (1993), Economics and Evolution: Bringing Life Back Into Economics, Oxford: Polity Press.
- Kauffman S. (1993), Origins of Order: Self-Organisation and Selection in Evolution, Oxford: Oxford University Press.
- Kauffman, Stuart (1993), The Origins of Order: Self-Organization and Selection in Evolution, Oxford University Press.
- Lucas R. E. (1978), Econometrica 46, 1429.
- Macy M.W., Willer R. (2002), 'From factors to actors: Computational sociology and agent-based modeling', Annual Review of Sociology 28: 143 – 166.
- Pugacheva E., Solovienko K. Self-Organization of Socio-Economic Systems, Irkutsk: BSUEL, 2003 (in Russian).
- Rothschild M. Bionomics: economy as ecosystem. New York: H.Holt, 1990. 423 p.
- The LSE Complexity Seminar “Complexity and Business Success”, October 1999
- Theory of Money, ed. Milton Friedman, Chicago,University of Chicago Press
- Yaneer Bar-Yam Market Failure: Interdependence in Action
The paper was published in:
- Pugacheva E. Complexity of Financial Crisis // Przeplywy kapitalu intelektualnego i finansowego - aspekty lokalne i globalne, Wyzsza Szkola Finansow i Zarzadzania w Bialymstoku, 2009, pp.151-164.
|
|